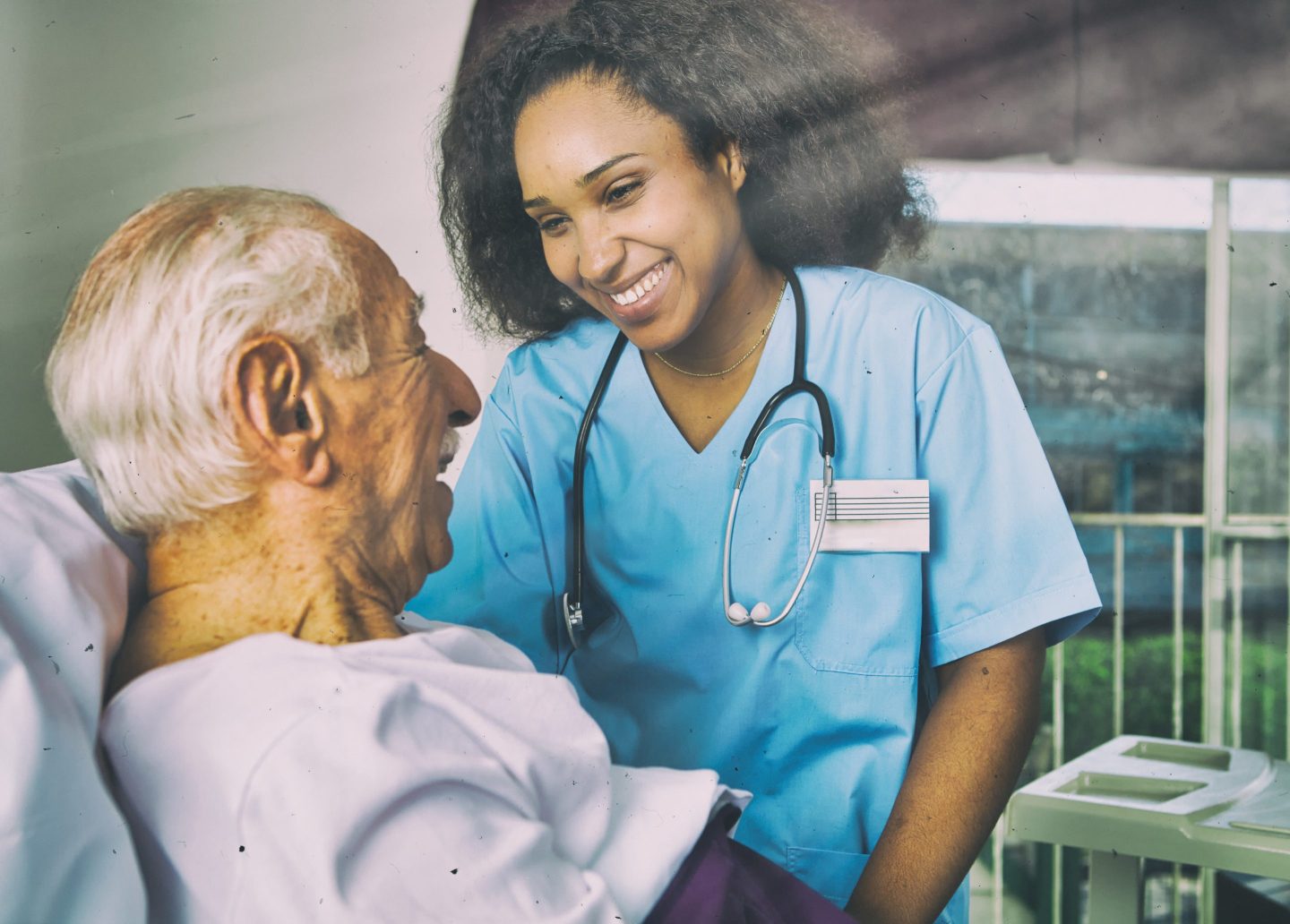
近年來,從電影制作到網(wǎng)絡(luò)安全,各行各業(yè)都掀起了一場(chǎng)人工智能革命。它甚至有可能在醫(yī)學(xué)領(lǐng)域突破一些困擾了科研人員幾十年的瓶頸問題。
最近幾年,人工智能在醫(yī)學(xué)領(lǐng)域的應(yīng)用日益廣泛,尤其是在疾病診斷方面。越來越多的醫(yī)生開始依賴深度學(xué)習(xí)技術(shù),這是一種模擬人腦運(yùn)行機(jī)制的機(jī)器學(xué)習(xí)技術(shù),而且具有較強(qiáng)的自主學(xué)習(xí)功能,因此能夠幫助我們檢測(cè)一些容易被忽略的嚴(yán)重健康問題,例如癌癥、心臟病等等,甚至也包括新冠病毒的無癥狀感染。
人工智能技術(shù)在醫(yī)學(xué)領(lǐng)域上的下一個(gè)突破性應(yīng)用,很可能是在阿爾茨海默氏癥的診斷上。這種疾病會(huì)導(dǎo)致不可逆的認(rèn)知下降和癡呆癥狀。人類發(fā)現(xiàn)阿爾茨海默氏癥已經(jīng)整整一個(gè)世紀(jì)了,但是迄今為止,我們尚未找到任何可靠的治療和早期檢測(cè)方法。
麻省總醫(yī)院(Massachusetts General Hospital)的研究人員最近測(cè)試了深度學(xué)習(xí)技術(shù)在阿爾茨海默氏癥檢測(cè)中的應(yīng)用,結(jié)果發(fā)現(xiàn),相比于其他沒有經(jīng)過多變量分析培訓(xùn)的人工智能模型,深度學(xué)習(xí)技術(shù)的檢測(cè)結(jié)果要更加準(zhǔn)確,即便不考慮一些容易產(chǎn)生誤導(dǎo)性的復(fù)雜因素(比如患者年齡),它也可能較精準(zhǔn)地診斷阿爾茨海默癥,相關(guān)發(fā)現(xiàn)已經(jīng)于上周發(fā)表在科研和醫(yī)學(xué)期刊《PLOS ONE》上。
在實(shí)驗(yàn)中,研究人員利用來自1萬多人的數(shù)萬張大腦掃描圖像,對(duì)一個(gè)深度學(xué)習(xí)模型進(jìn)行了訓(xùn)練。這些人有的患有阿爾茨海默癥,有的則沒有。然后研究人員將人工智能模型的診斷結(jié)果與真實(shí)臨床數(shù)據(jù)進(jìn)行了比對(duì)測(cè)試。
實(shí)驗(yàn)結(jié)果顯示,深度學(xué)習(xí)模型診斷阿爾茨海默癥的準(zhǔn)確率達(dá)到了90.2%,比不依賴深度學(xué)習(xí)技術(shù)的簡單人工智能模型高出了大約5個(gè)百分點(diǎn)。不論患者被診斷的時(shí)間地點(diǎn),也不管他們的年紀(jì)多大,深度學(xué)習(xí)人工智能的診斷結(jié)果都表現(xiàn)得更出色。
麻省總醫(yī)院的研究員、該論文的主要作者馬修·萊明在一份聲明里指出:“這是目前僅有的定期收集大腦核磁共振圖像(MRI)用于老年癡呆癥診斷的研究之一。我們的研究結(jié)果具有跨地區(qū)、跨時(shí)間和跨人群的普遍性,因此為這種診斷技術(shù)的臨床應(yīng)用提供了強(qiáng)有力的支持?!?/p>
阿爾茨海默癥的診斷歷來是有一定難度的,人工智能模型90%的診斷準(zhǔn)確率,已經(jīng)遠(yuǎn)遠(yuǎn)高于人類醫(yī)生的臨床診斷準(zhǔn)確率。根據(jù)2017年的一項(xiàng)研究,人類醫(yī)生對(duì)阿爾茨海默癥的臨床診斷準(zhǔn)確率約為77%。
人工智能在醫(yī)學(xué)領(lǐng)域的廣泛應(yīng)用前景
最近隨著ChatGPT的走紅,OpenAI、微軟(Microsoft)和谷歌(Google)等公司開發(fā)的人工智能應(yīng)用成了人們談?wù)摰臒狳c(diǎn),因?yàn)樗鼈兒苡锌赡茴嵏菜阉餍袠I(yè),甚至徹底改變我們的工作方式。而機(jī)器學(xué)習(xí)技術(shù)則在醫(yī)學(xué)領(lǐng)域擁有拯救生命的潛力。
美國衛(wèi)生與公眾服務(wù)部(Department of Health and Human Services)2022年12月的一項(xiàng)研究顯示,美國每年在醫(yī)院被誤診的人數(shù)高達(dá)700萬人。近300萬急診患者因?yàn)檎`診而遭受不良后果,超過37萬人因?yàn)檎`診而導(dǎo)致永久性殘疾甚至死亡。
一個(gè)名為“改善醫(yī)療診斷協(xié)會(huì)”(Society to Improve Diagnosis in Medicine)的非盈利機(jī)構(gòu)指出,誤診還會(huì)給消費(fèi)者帶來沉重的經(jīng)濟(jì)負(fù)擔(dān)。如果解決了誤診問題,以及由誤診引發(fā)的醫(yī)療訴訟,每年就可以節(jié)省大約1,000億美元。
廣大醫(yī)生都表示,人工智能在改善診斷技術(shù)方面擁有巨大潛能,盡管有些地方也出現(xiàn)了不少與人工智能技術(shù)相關(guān)的問題——例如事實(shí)性錯(cuò)誤和種族歧視等等。2022年發(fā)表的一篇關(guān)于人工智能在醫(yī)療診斷方面的文獻(xiàn)綜述發(fā)現(xiàn),人工智能技術(shù)在癌癥、糖尿病和阿爾茨海默癥的診斷上都很有前景,只是要想提高人工智能診斷的準(zhǔn)確率,還需要我們進(jìn)行進(jìn)一步的研究。
人工智能在阿爾茨海默癥診斷中扮演的重要角色
如果正如我們所預(yù)料的那樣,人工智能和機(jī)器學(xué)習(xí)在醫(yī)學(xué)診斷上得到了更廣泛的應(yīng)用,那么它首先造福的就是阿爾茨海默癥患者,因?yàn)檫@種疾病被醫(yī)學(xué)界公認(rèn)為是最難預(yù)測(cè)和診斷的疾病之一。
阿爾茨海默癥是老年人中最常見的一種老年癡呆癥,全世界大約有4,400萬名阿爾茨海默癥患者。不過老年癡呆的癥狀可能與很多癥狀有關(guān),阿爾茨海默癥只是導(dǎo)致癡呆的病因之一,還有很多癥狀也很容易被誤診為阿爾茨海默癥。
2017年的一項(xiàng)針對(duì)900多人的研究發(fā)現(xiàn),多達(dá)四分之一的阿爾茨海默病患者曾經(jīng)被誤診,其中假陽性和假陰性的比例大致相同。阿爾茨海默病之所以容易被誤診,是因?yàn)樗陌Y狀與一些常見的神經(jīng)系統(tǒng)疾病很像,比如路易體癡呆、額顳葉癡呆等。美國神經(jīng)病學(xué)學(xué)會(huì)(American Academy of Neurology)指出,阿爾茨海默癥的誤診機(jī)率與年齡呈正相關(guān),也就是說阿爾茨海默癥與其他類型的癡呆癥“在老年人中很容易被誤診”。
預(yù)測(cè)一個(gè)病人會(huì)不會(huì)患上阿爾茨海默癥,并不比診斷這種疾病更容易,因?yàn)?0%的阿爾茨海默癥病例是“偶發(fā)性”的——也就是病人通常并沒有家族病史。由于存在這些困難因素,阿爾茨海默癥幾乎沒有可靠的早期篩查模型,大多數(shù)病例都是在腦損傷癥狀出現(xiàn)后,才被診斷出來的。
麻省總醫(yī)院的研究沒有涉及深度學(xué)習(xí)技術(shù)是否能夠用于阿爾茨海默癥的預(yù)測(cè)上,但其他一些研究似乎表明,人工智能是很有可能在這方面發(fā)揮重要作用的。
例如佛羅里達(dá)大學(xué)(University of Florida)在上周宣布,該大學(xué)開發(fā)的人工智能模型可以通過研究患者的電子病歷,提前五年預(yù)測(cè)哪些人患阿爾茨海默癥的風(fēng)險(xiǎn)較高。雖然研究人員建議,一線醫(yī)生在使用人工智能工具進(jìn)行預(yù)測(cè)前,應(yīng)該對(duì)其進(jìn)行更多的測(cè)試,但他們也發(fā)現(xiàn),人工智能模型能夠有效用于早期診斷,因此從長期來看可以降低疾病的嚴(yán)重程度。(財(cái)富中文網(wǎng))
譯者:樸成奎
近年來,從電影制作到網(wǎng)絡(luò)安全,各行各業(yè)都掀起了一場(chǎng)人工智能革命。它甚至有可能在醫(yī)學(xué)領(lǐng)域突破一些困擾了科研人員幾十年的瓶頸問題。
最近幾年,人工智能在醫(yī)學(xué)領(lǐng)域的應(yīng)用日益廣泛,尤其是在疾病診斷方面。越來越多的醫(yī)生開始依賴深度學(xué)習(xí)技術(shù),這是一種模擬人腦運(yùn)行機(jī)制的機(jī)器學(xué)習(xí)技術(shù),而且具有較強(qiáng)的自主學(xué)習(xí)功能,因此能夠幫助我們檢測(cè)一些容易被忽略的嚴(yán)重健康問題,例如癌癥、心臟病等等,甚至也包括新冠病毒的無癥狀感染。
人工智能技術(shù)在醫(yī)學(xué)領(lǐng)域上的下一個(gè)突破性應(yīng)用,很可能是在阿爾茨海默氏癥的診斷上。這種疾病會(huì)導(dǎo)致不可逆的認(rèn)知下降和癡呆癥狀。人類發(fā)現(xiàn)阿爾茨海默氏癥已經(jīng)整整一個(gè)世紀(jì)了,但是迄今為止,我們尚未找到任何可靠的治療和早期檢測(cè)方法。
麻省總醫(yī)院(Massachusetts General Hospital)的研究人員最近測(cè)試了深度學(xué)習(xí)技術(shù)在阿爾茨海默氏癥檢測(cè)中的應(yīng)用,結(jié)果發(fā)現(xiàn),相比于其他沒有經(jīng)過多變量分析培訓(xùn)的人工智能模型,深度學(xué)習(xí)技術(shù)的檢測(cè)結(jié)果要更加準(zhǔn)確,即便不考慮一些容易產(chǎn)生誤導(dǎo)性的復(fù)雜因素(比如患者年齡),它也可能較精準(zhǔn)地診斷阿爾茨海默癥,相關(guān)發(fā)現(xiàn)已經(jīng)于上周發(fā)表在科研和醫(yī)學(xué)期刊《PLOS ONE》上。
在實(shí)驗(yàn)中,研究人員利用來自1萬多人的數(shù)萬張大腦掃描圖像,對(duì)一個(gè)深度學(xué)習(xí)模型進(jìn)行了訓(xùn)練。這些人有的患有阿爾茨海默癥,有的則沒有。然后研究人員將人工智能模型的診斷結(jié)果與真實(shí)臨床數(shù)據(jù)進(jìn)行了比對(duì)測(cè)試。
實(shí)驗(yàn)結(jié)果顯示,深度學(xué)習(xí)模型診斷阿爾茨海默癥的準(zhǔn)確率達(dá)到了90.2%,比不依賴深度學(xué)習(xí)技術(shù)的簡單人工智能模型高出了大約5個(gè)百分點(diǎn)。不論患者被診斷的時(shí)間地點(diǎn),也不管他們的年紀(jì)多大,深度學(xué)習(xí)人工智能的診斷結(jié)果都表現(xiàn)得更出色。
麻省總醫(yī)院的研究員、該論文的主要作者馬修·萊明在一份聲明里指出:“這是目前僅有的定期收集大腦核磁共振圖像(MRI)用于老年癡呆癥診斷的研究之一。我們的研究結(jié)果具有跨地區(qū)、跨時(shí)間和跨人群的普遍性,因此為這種診斷技術(shù)的臨床應(yīng)用提供了強(qiáng)有力的支持。”
阿爾茨海默癥的診斷歷來是有一定難度的,人工智能模型90%的診斷準(zhǔn)確率,已經(jīng)遠(yuǎn)遠(yuǎn)高于人類醫(yī)生的臨床診斷準(zhǔn)確率。根據(jù)2017年的一項(xiàng)研究,人類醫(yī)生對(duì)阿爾茨海默癥的臨床診斷準(zhǔn)確率約為77%。
人工智能在醫(yī)學(xué)領(lǐng)域的廣泛應(yīng)用前景
最近隨著ChatGPT的走紅,OpenAI、微軟(Microsoft)和谷歌(Google)等公司開發(fā)的人工智能應(yīng)用成了人們談?wù)摰臒狳c(diǎn),因?yàn)樗鼈兒苡锌赡茴嵏菜阉餍袠I(yè),甚至徹底改變我們的工作方式。而機(jī)器學(xué)習(xí)技術(shù)則在醫(yī)學(xué)領(lǐng)域擁有拯救生命的潛力。
美國衛(wèi)生與公眾服務(wù)部(Department of Health and Human Services)2022年12月的一項(xiàng)研究顯示,美國每年在醫(yī)院被誤診的人數(shù)高達(dá)700萬人。近300萬急診患者因?yàn)檎`診而遭受不良后果,超過37萬人因?yàn)檎`診而導(dǎo)致永久性殘疾甚至死亡。
一個(gè)名為“改善醫(yī)療診斷協(xié)會(huì)”(Society to Improve Diagnosis in Medicine)的非盈利機(jī)構(gòu)指出,誤診還會(huì)給消費(fèi)者帶來沉重的經(jīng)濟(jì)負(fù)擔(dān)。如果解決了誤診問題,以及由誤診引發(fā)的醫(yī)療訴訟,每年就可以節(jié)省大約1,000億美元。
廣大醫(yī)生都表示,人工智能在改善診斷技術(shù)方面擁有巨大潛能,盡管有些地方也出現(xiàn)了不少與人工智能技術(shù)相關(guān)的問題——例如事實(shí)性錯(cuò)誤和種族歧視等等。2022年發(fā)表的一篇關(guān)于人工智能在醫(yī)療診斷方面的文獻(xiàn)綜述發(fā)現(xiàn),人工智能技術(shù)在癌癥、糖尿病和阿爾茨海默癥的診斷上都很有前景,只是要想提高人工智能診斷的準(zhǔn)確率,還需要我們進(jìn)行進(jìn)一步的研究。
人工智能在阿爾茨海默癥診斷中扮演的重要角色
如果正如我們所預(yù)料的那樣,人工智能和機(jī)器學(xué)習(xí)在醫(yī)學(xué)診斷上得到了更廣泛的應(yīng)用,那么它首先造福的就是阿爾茨海默癥患者,因?yàn)檫@種疾病被醫(yī)學(xué)界公認(rèn)為是最難預(yù)測(cè)和診斷的疾病之一。
阿爾茨海默癥是老年人中最常見的一種老年癡呆癥,全世界大約有4,400萬名阿爾茨海默癥患者。不過老年癡呆的癥狀可能與很多癥狀有關(guān),阿爾茨海默癥只是導(dǎo)致癡呆的病因之一,還有很多癥狀也很容易被誤診為阿爾茨海默癥。
2017年的一項(xiàng)針對(duì)900多人的研究發(fā)現(xiàn),多達(dá)四分之一的阿爾茨海默病患者曾經(jīng)被誤診,其中假陽性和假陰性的比例大致相同。阿爾茨海默病之所以容易被誤診,是因?yàn)樗陌Y狀與一些常見的神經(jīng)系統(tǒng)疾病很像,比如路易體癡呆、額顳葉癡呆等。美國神經(jīng)病學(xué)學(xué)會(huì)(American Academy of Neurology)指出,阿爾茨海默癥的誤診機(jī)率與年齡呈正相關(guān),也就是說阿爾茨海默癥與其他類型的癡呆癥“在老年人中很容易被誤診”。
預(yù)測(cè)一個(gè)病人會(huì)不會(huì)患上阿爾茨海默癥,并不比診斷這種疾病更容易,因?yàn)?0%的阿爾茨海默癥病例是“偶發(fā)性”的——也就是病人通常并沒有家族病史。由于存在這些困難因素,阿爾茨海默癥幾乎沒有可靠的早期篩查模型,大多數(shù)病例都是在腦損傷癥狀出現(xiàn)后,才被診斷出來的。
麻省總醫(yī)院的研究沒有涉及深度學(xué)習(xí)技術(shù)是否能夠用于阿爾茨海默癥的預(yù)測(cè)上,但其他一些研究似乎表明,人工智能是很有可能在這方面發(fā)揮重要作用的。
例如佛羅里達(dá)大學(xué)(University of Florida)在上周宣布,該大學(xué)開發(fā)的人工智能模型可以通過研究患者的電子病歷,提前五年預(yù)測(cè)哪些人患阿爾茨海默癥的風(fēng)險(xiǎn)較高。雖然研究人員建議,一線醫(yī)生在使用人工智能工具進(jìn)行預(yù)測(cè)前,應(yīng)該對(duì)其進(jìn)行更多的測(cè)試,但他們也發(fā)現(xiàn),人工智能模型能夠有效用于早期診斷,因此從長期來看可以降低疾病的嚴(yán)重程度。(財(cái)富中文網(wǎng))
譯者:樸成奎
Artificial intelligence is already revolutionizing everything from filmmaking to cybersecurity, and it could also be poised to create major breakthroughs in medicine that have stumped researchers for decades.
The use of A.I. in medicine has been growing in recent years, especially in diagnosing illnesses and diseases. A growing number of doctors already rely on deep learning, a machine learning method modeled on artificial neural networks to learn by example as human brains do, to help detect potentially life-threatening conditions that can be easily missed, such as cancer, heart disease, and even asymptomatic cases of COVID-19.
But the next breakthrough for A.I. in medicine could be in identifying Alzheimer’s, the devastating ailment that causes irreversible cognitive decline and dementia, for which treatment and reliable early detection have eluded medical researchers in the century since the disease’s discovery.
Researchers at Massachusetts General Hospital recently tested deep learning techniques in Alzheimer’s detection, and found that not only was deep learning more accurate than comparative A.I. models that weren’t trained to analyze multiple variables together, it was also able to identify Alzheimer’s cases regardless of factors that usually complicate early-onset detection, such as a patient’s age. The findings were reported in a study published last week in PLOS ONE, a scientific and medical journal.
The researchers trained a deep learning model with tens of thousands of brain scan images collected from over 10,000 people, both with and without Alzheimer’s disease. The study then tested the model against real-world clinical data of Alzheimer’s diagnoses.
The deep learning model was able to identify Alzheimer’s cases with a 90.2% accuracy rate, around five percentage points higher than the simpler A.I. models that did not rely on the deep learning system. The A.I. model performed better regardless of when and where patients were diagnosed with Alzheimer’s, as well as how old they were at the time.
“This is one of the only studies that used routinely collected brain MRIs to attempt to detect dementia,” Matthew Leming, a research fellow at Massachusetts General Hospital and lead author on the study, said in a statement. “Our results—with cross-site, cross-time, and cross-population generalizability—make a strong case for clinical use of this diagnostic technology.”
A 90% accuracy rate in Alzheimer’s diagnosis would be leaps and bounds ahead of human clinical detection rates, which, according to a 2017 study, stand at 77%.
A.I.’s big medical splash
While A.I.-powered search engines developed by OpenAI, Microsoft, and Google have grabbed most of the headlines about artificial intelligence recently for how they promise to disrupt search and how we work, machine learning could have potentially lifesaving applications in medicine.
More than 7 million people admitted to U.S. emergency rooms every year are diagnosed incorrectly, according to a December study by the Department of Health and Human Services. That study found that almost 3 million ER patients are saddled with adverse effects from a misdiagnosis, while over 370,000 suffer from a permanent disability or death.
Misdiagnosis is an economic burden too, as eliminating incorrect testing and treatments as well as the malpractice lawsuits stemming from misdiagnoses could add up to around $100 billion a year in savings, according to the Society to Improve Diagnosis in Medicine, a nonprofit.
Doctors and physicians have said that A.I. holds significant promise in efforts to improve diagnostic techniques, although many of the same issues with A.I. that have been found elsewhere, such as the potential for factual mistakes and racial biases, have also cropped up in medical research. A literature review of A.I. in medical diagnosis published last year found that the technology has promise in fields including cancer, diabetes, and Alzheimer’s diagnosis, although further research is recommended to improve A.I.’s accuracy in identifying medical issues.
A big role in Alzheimer’s research
But if future research makes A.I. and deep learning more widely used in diagnosis, it may be a game changer for Alzheimer’s, which is one of most difficult diseases to predict and diagnose.
Alzheimer’s is the most common type of dementia among older people, afflicting around 44 million worldwide. But it is only one form of a large family of dementia-related conditions, which can easily be misinterpreted as Alzheimer’s.
A 2017 study of over 900 people found that up to one in four Alzheimer’s patients were misdiagnosed, with a roughly even split between false positives and false negatives. Alzheimer’s proclivity for misdiagnosis largely comes down to how many of its symptoms overlap with other common neurological disorders, including Lewy body or frontotemporal dementia. The chances of misdiagnosis increase with age, according to the American Academy of Neurology, which says that Alzheimer’s disease and other dementing illnesses “may be easily misdiagnosed in the elderly.”
Predicting a patient will come down with Alzheimer’s is no easier than diagnosing it, as over 90% of Alzheimer’s cases are considered “sporadic”—appearing in patients with no family link to the disease. Because of these difficulties, there are almost no reliable early screening models for Alzheimer’s, with most cases being diagnosed after symptoms of brain damage begin to be seen.
Massachusetts General Hospital’s study did not address whether deep learning could help with predicting Alzheimer’s, but other studies seem to suggest A.I. could have an important role to play there too.
An A.I. model developed at the University of Florida was able to tap into electronic health records to predict which patients were at a high risk of developing Alzheimer’s up to five years before a diagnosis, the university announced last week. While the researchers recommended more testing before doctors begin employing A.I. predicting tools, they found that A.I. models could help with early diagnosis and reduce the severity of the disease in the long term.