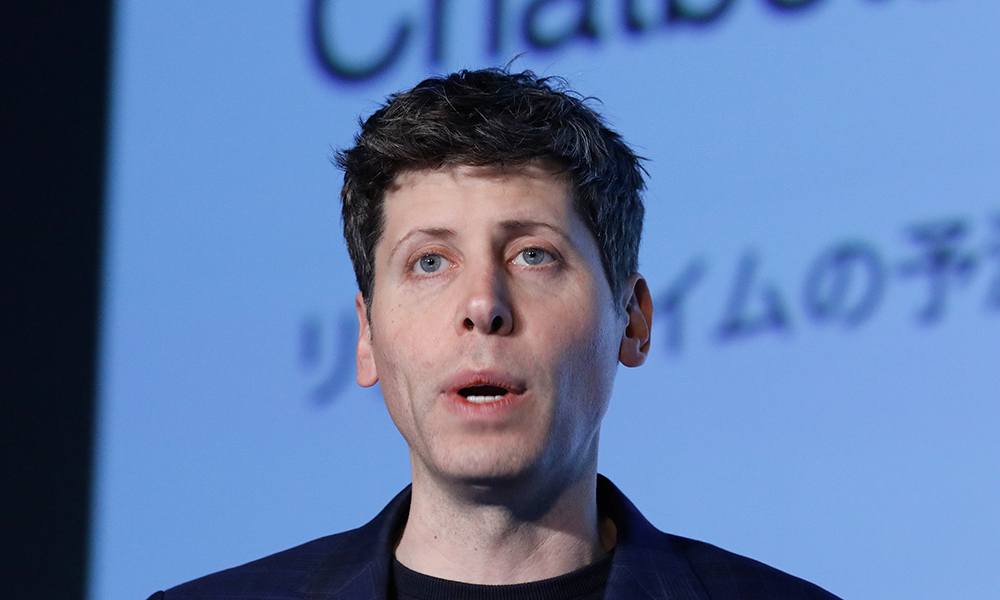
薩姆·奧爾特曼最近宣稱,OpenAI已經(jīng)知道如何構(gòu)建通用人工智能(AGI),這再次引發(fā)了有關(guān)AI未來的辯論。雖然這些主張頻頻登上媒體頭條,但作為一名研究人腦工作原理超過十年的神經(jīng)科學家,我發(fā)現(xiàn)自己關(guān)注的是一個不同的悖論:對現(xiàn)代AI系統(tǒng)最常見的批評之一是我們沒有完全理解它們的工作原理,這實際上可能是它們最像人腦的特征之一。
當前的AI熱潮導(dǎo)致對AGI有各種模糊的定義。但從神經(jīng)科學的角度來看待AI,為我們提供了寶貴的機會,對AI的能力和局限性進行有意義的現(xiàn)實檢驗。
現(xiàn)實是,盡管經(jīng)過了幾個世紀的科學探究,我們?nèi)匀粵]有完全了解人類大腦的工作機制。作為研究人員,我們可以觀察到某些神經(jīng)元執(zhí)行特定功能,但關(guān)于我們的認知過程實際上如何執(zhí)行,這種知識的解釋能力極其有限。然而,這并沒有阻止我們成為有生產(chǎn)能力的社會成員或者做出重要的決策。
同樣,我們理解AI背后的數(shù)學原理,但在相對簡單的數(shù)學運算和這些系統(tǒng)表現(xiàn)出的非凡智能之間存在著神奇的飛躍。這種生物神經(jīng)網(wǎng)絡(luò)和人工神經(jīng)網(wǎng)絡(luò)之間的相似性不是缺陷,而是復(fù)雜智能系統(tǒng)的標志。
以我和朋友最近使用OpenAI o1(目前最先進的 AI 模型之一)的經(jīng)歷為例。我們向它展示了一個由不同州的車牌拼接而成的視覺謎題,并要求它識別每個字母或數(shù)字來自哪個州。經(jīng)過幾分鐘的思考,它提供了一段清晰流暢而自信的分析,但結(jié)果幾乎完全錯誤。
即使在收到詳細反饋之后,它又生成了一個自信但同樣錯誤的答案。這暴露出AI的一個關(guān)鍵局限性:盡管當前的AI在許多領(lǐng)域表現(xiàn)出色,但它可能缺乏自我意識,無法識別它可能出錯的情況,而這是人類智能的一個寶貴元素。
商業(yè)意義
這揭示了一個關(guān)于智能的更廣泛的真相:智能不是一種單一的能力,而是一系列專門學習系統(tǒng)的組合。人腦有不同的機制——從語義記憶(如知道2+2=4的事實知識)到回憶個人體驗的情景記憶(如記住你第一次學習算術(shù)的時刻),以及隱性概率學習(如在不自覺理解原因的情況下提高網(wǎng)球水平)。盡管AI基準測試變得越來越全面和嚴格,但它們?nèi)晕茨懿蹲降饺祟愔悄艿娜慷鄻有浴?/p>
對于商業(yè)領(lǐng)袖而言,這具有重要意義。當前的AI浪潮并不是要全面取代人類智能,而是要理解這些工具可以在哪些方面補充人類的能力——以及在哪些方面無法作為補充。例如,在創(chuàng)意領(lǐng)域,生成式AI尚無法生成比人類專業(yè)人士更好的圖像或視頻。它是一種需要人類監(jiān)督的“人機協(xié)同”工具。
隨著AI能力的增長,這種理解變得日益重要。特斯拉(Tesla)的自動駕駛技術(shù)展示了其未來前景和風險:盡管它可能在99.9%的時間內(nèi)表現(xiàn)出色,但人類往往難以辨別99.9%和99.9999%準確率之間的差異。因此,我們可能對其產(chǎn)生一種過度的信任,忽視了小數(shù)點后增加幾位數(shù)對于確保真正安全的重要性。偶爾出現(xiàn)的不可預(yù)測的故障會提醒人們,這些系統(tǒng)尚未完全符合人類預(yù)期的復(fù)雜性和現(xiàn)實世界的不可預(yù)測性。
人工智能的局限性
雖然機器在長期記憶和處理速度方面早已超越了人類的能力,現(xiàn)在在其他領(lǐng)域似乎也將超越人類,但相比一些行業(yè)領(lǐng)導(dǎo)者的觀點,全面復(fù)制人類的智能仍然是一個更遙不可及的目標。值得注意的是,大多數(shù)AI基準測試將機器性能與人類個體進行比較。然而,人類通常不是孤立的。從建立文明到解碼人類基因組,人類最重要的成就都是集體努力和合作的產(chǎn)物。共享知識、團隊合作和文化傳遞的專業(yè)知識使我們能夠突破個人的局限性。因此,雖然AI模型可能在某些任務(wù)上優(yōu)于人類個體,但它并不能復(fù)制一群人共同工作時所產(chǎn)生的那種協(xié)同智能。這種由語言、文化和社會互動推動的動態(tài)、集體問題解決能力是人類智能的一個關(guān)鍵方面,當前的AI系統(tǒng)并不完全具備這種能力。
理解這種更細微的現(xiàn)實,對于公司高管應(yīng)用AI至關(guān)重要。我們不能局限于AI是否達到了人類智能水平這個二元問題,而是應(yīng)該專注于理解AI系統(tǒng)在具體維度上的優(yōu)勢和劣勢。成功采用AI的關(guān)鍵不是盲目信任或全面懷疑,而是要細致地理解這些系統(tǒng)的能力和局限性。正如我們在不完全理解人類智能的情況下學會了高效利用人類智能一樣,我們需要開發(fā)與人工智能合作的框架,既承認其非凡的能力,也承認其固有的不可預(yù)測性。
這并不意味著要放慢開發(fā)AI的速度,其實AI的進開發(fā)展令人驚嘆,值得慶祝。但隨著這些系統(tǒng)變得越來越復(fù)雜,且對AGI的辯論仍在繼續(xù),我們就需要保持一種平衡的觀點,既認識到它們的變革潛力,也認識到它們的基本局限性。AI的未來不是實現(xiàn)完美的理解或控制,而是學會如何與這些系統(tǒng)有效合作,這些系統(tǒng)可能像我們自己的大腦一樣,總是保留著某種神秘元素。(財富中文網(wǎng))
本文作者亞倫·安達爾曼現(xiàn)任Cognitiv的首席科學家兼聯(lián)合創(chuàng)始人。
Fortune.com上發(fā)表的評論文章中表達的觀點,僅代表作者本人的觀點,不能代表《財富》雜志的觀點和立場。
譯者:劉進龍
審校:汪皓
薩姆·奧爾特曼最近宣稱,OpenAI已經(jīng)知道如何構(gòu)建通用人工智能(AGI),這再次引發(fā)了有關(guān)AI未來的辯論。雖然這些主張頻頻登上媒體頭條,但作為一名研究人腦工作原理超過十年的神經(jīng)科學家,我發(fā)現(xiàn)自己關(guān)注的是一個不同的悖論:對現(xiàn)代AI系統(tǒng)最常見的批評之一是我們沒有完全理解它們的工作原理,這實際上可能是它們最像人腦的特征之一。
當前的AI熱潮導(dǎo)致對AGI有各種模糊的定義。但從神經(jīng)科學的角度來看待AI,為我們提供了寶貴的機會,對AI的能力和局限性進行有意義的現(xiàn)實檢驗。
現(xiàn)實是,盡管經(jīng)過了幾個世紀的科學探究,我們?nèi)匀粵]有完全了解人類大腦的工作機制。作為研究人員,我們可以觀察到某些神經(jīng)元執(zhí)行特定功能,但關(guān)于我們的認知過程實際上如何執(zhí)行,這種知識的解釋能力極其有限。然而,這并沒有阻止我們成為有生產(chǎn)能力的社會成員或者做出重要的決策。
同樣,我們理解AI背后的數(shù)學原理,但在相對簡單的數(shù)學運算和這些系統(tǒng)表現(xiàn)出的非凡智能之間存在著神奇的飛躍。這種生物神經(jīng)網(wǎng)絡(luò)和人工神經(jīng)網(wǎng)絡(luò)之間的相似性不是缺陷,而是復(fù)雜智能系統(tǒng)的標志。
以我和朋友最近使用OpenAI o1(目前最先進的 AI 模型之一)的經(jīng)歷為例。我們向它展示了一個由不同州的車牌拼接而成的視覺謎題,并要求它識別每個字母或數(shù)字來自哪個州。經(jīng)過幾分鐘的思考,它提供了一段清晰流暢而自信的分析,但結(jié)果幾乎完全錯誤。
即使在收到詳細反饋之后,它又生成了一個自信但同樣錯誤的答案。這暴露出AI的一個關(guān)鍵局限性:盡管當前的AI在許多領(lǐng)域表現(xiàn)出色,但它可能缺乏自我意識,無法識別它可能出錯的情況,而這是人類智能的一個寶貴元素。
商業(yè)意義
這揭示了一個關(guān)于智能的更廣泛的真相:智能不是一種單一的能力,而是一系列專門學習系統(tǒng)的組合。人腦有不同的機制——從語義記憶(如知道2+2=4的事實知識)到回憶個人體驗的情景記憶(如記住你第一次學習算術(shù)的時刻),以及隱性概率學習(如在不自覺理解原因的情況下提高網(wǎng)球水平)。盡管AI基準測試變得越來越全面和嚴格,但它們?nèi)晕茨懿蹲降饺祟愔悄艿娜慷鄻有浴?/p>
對于商業(yè)領(lǐng)袖而言,這具有重要意義。當前的AI浪潮并不是要全面取代人類智能,而是要理解這些工具可以在哪些方面補充人類的能力——以及在哪些方面無法作為補充。例如,在創(chuàng)意領(lǐng)域,生成式AI尚無法生成比人類專業(yè)人士更好的圖像或視頻。它是一種需要人類監(jiān)督的“人機協(xié)同”工具。
隨著AI能力的增長,這種理解變得日益重要。特斯拉(Tesla)的自動駕駛技術(shù)展示了其未來前景和風險:盡管它可能在99.9%的時間內(nèi)表現(xiàn)出色,但人類往往難以辨別99.9%和99.9999%準確率之間的差異。因此,我們可能對其產(chǎn)生一種過度的信任,忽視了小數(shù)點后增加幾位數(shù)對于確保真正安全的重要性。偶爾出現(xiàn)的不可預(yù)測的故障會提醒人們,這些系統(tǒng)尚未完全符合人類預(yù)期的復(fù)雜性和現(xiàn)實世界的不可預(yù)測性。
人工智能的局限性
雖然機器在長期記憶和處理速度方面早已超越了人類的能力,現(xiàn)在在其他領(lǐng)域似乎也將超越人類,但相比一些行業(yè)領(lǐng)導(dǎo)者的觀點,全面復(fù)制人類的智能仍然是一個更遙不可及的目標。值得注意的是,大多數(shù)AI基準測試將機器性能與人類個體進行比較。然而,人類通常不是孤立的。從建立文明到解碼人類基因組,人類最重要的成就都是集體努力和合作的產(chǎn)物。共享知識、團隊合作和文化傳遞的專業(yè)知識使我們能夠突破個人的局限性。因此,雖然AI模型可能在某些任務(wù)上優(yōu)于人類個體,但它并不能復(fù)制一群人共同工作時所產(chǎn)生的那種協(xié)同智能。這種由語言、文化和社會互動推動的動態(tài)、集體問題解決能力是人類智能的一個關(guān)鍵方面,當前的AI系統(tǒng)并不完全具備這種能力。
理解這種更細微的現(xiàn)實,對于公司高管應(yīng)用AI至關(guān)重要。我們不能局限于AI是否達到了人類智能水平這個二元問題,而是應(yīng)該專注于理解AI系統(tǒng)在具體維度上的優(yōu)勢和劣勢。成功采用AI的關(guān)鍵不是盲目信任或全面懷疑,而是要細致地理解這些系統(tǒng)的能力和局限性。正如我們在不完全理解人類智能的情況下學會了高效利用人類智能一樣,我們需要開發(fā)與人工智能合作的框架,既承認其非凡的能力,也承認其固有的不可預(yù)測性。
這并不意味著要放慢開發(fā)AI的速度,其實AI的進開發(fā)展令人驚嘆,值得慶祝。但隨著這些系統(tǒng)變得越來越復(fù)雜,且對AGI的辯論仍在繼續(xù),我們就需要保持一種平衡的觀點,既認識到它們的變革潛力,也認識到它們的基本局限性。AI的未來不是實現(xiàn)完美的理解或控制,而是學會如何與這些系統(tǒng)有效合作,這些系統(tǒng)可能像我們自己的大腦一樣,總是保留著某種神秘元素。(財富中文網(wǎng))
本文作者亞倫·安達爾曼現(xiàn)任Cognitiv的首席科學家兼聯(lián)合創(chuàng)始人。
Fortune.com上發(fā)表的評論文章中表達的觀點,僅代表作者本人的觀點,不能代表《財富》雜志的觀點和立場。
譯者:劉進龍
審校:汪皓
Sam Altman’s recent declaration that OpenAI now knows how to build artificial general intelligence (AGI) has reignited debates about AI’s future. While such claims generate headlines, as a neuroscientist who spent over a decade studying how the brain works, I find myself focused on a different paradox: One of the most common criticisms of modern AI systems—that we do not fully understand how they work—might actually be one of their most brain-like features.
The current AI hype cycle has led to varied, ambiguous definitions of AGI. But looking at AI through the lens of neuroscience offers a valuable reality check about both its capabilities and limitations.
The reality is that despite centuries of scientific inquiry, we still do not fully understand how the human brain works. As researchers, we can observe that certain neurons perform specific functions, but this knowledge offers limited explanatory power about how our cognitive processes actually function. Yet this has not stopped us from being productive members of society or making important decisions.
Similarly, we understand the mathematics behind AI, but there is a mysterious leap between these relatively simple mathematical operations and the remarkable intelligence these systems display. This parallel between biological and artificial neural networks is not a flaw—it is a signature of complex intelligence systems.
Consider a recent experience my friend and I had with OpenAI o1, one of the most advanced AI models out there. We presented it with a visual puzzle of spliced license plates from different states and asked it to identify the origin state of each individual letter or number. After thinking for several minutes, it provided a beautifully articulated and confident analysis that was nearly completely incorrect.
Even after detailed feedback, it produced another confident, but equally wrong, answer. This reveals one of AI’s crucial limitations: Despite impressive capabilities in many areas, today’s AI can lack the self-awareness to recognize when it might be wrong, which is a valuable element of human intelligence.
Business implications
This points to a broader truth about intelligence: It is not a monolithic capability but rather a tapestry of specialized learning systems. Our brains have distinct mechanisms—from semantic memory for factual knowledge (like knowing that 2+2=4) to episodic memory for recalling personal experiences (like remembering the moment you first learned arithmetic), and implicit probabilistic learning (like improving at tennis without consciously understanding why). While AI benchmarks become increasingly comprehensive and rigorous, they still do not capture this full diversity of human intelligence.
For business leaders, this has important implications. The current wave of AI is not about replacing human intelligence wholesale, but about understanding where these tools can complement human capabilities—and where they cannot. In creative fields, for instance, generative AI is not yet capable of producing better images or videos than human professionals. It is a tool that needs to remain “in the loop” with human oversight.
The stakes of this understanding grow higher as AI capabilities grow. Tesla’s self-driving technology demonstrates both the promise and the peril: While it may perform impressively 99.9% of the time, humans often have difficulty discerning the difference between 99.9% and 99.9999% accuracy. As a result, we risk developing an undue level of trust that overlooks how vital those extra nines are for ensuring true safety. The occasional unpredictable failure thus serves as a stark reminder that these systems are not yet fully aligned with the complexities of human expectations and real-world unpredictability.
AI limitations
While machines surpassed human capabilities in long-term memory and processing speed long ago and now appear poised to exceed us in other domains, replicating the full breadth of human intelligence remains a far more elusive goal than some industry leaders suggest. It is worth noting that most AI benchmarks compare machine performance to individual humans. Yet humans do not generally operate in isolation. Our species’ most significant achievements—from building civilizations to decoding the human genome—are products of collective effort and collaboration. Shared knowledge, teamwork, and culturally transmitted expertise allow us to transcend our individual limitations. So while an AI model might outperform a lone human on certain tasks, it does not replicate the kind of collaborative intelligence that emerges when groups of people work together. This capacity for dynamic, collective problem-solving—fueled by language, culture, and social interaction—is a key aspect of human intelligence that current AI systems do not fully capture.
Understanding this more nuanced reality is crucial for executives navigating the AI landscape—we need to move beyond the binary question of whether AI has reached human-level intelligence and instead focus on understanding the specific dimensions along which AI systems excel or fall short. The key to successful AI implementation is not blind trust or wholesale skepticism, but rather a nuanced understanding of these systems’ capabilities and limitations. Just as we have learned to work productively with human intelligence despite not fully understanding it, we need to develop frameworks for working with artificial intelligence that acknowledge both its remarkable capabilities and its inherent unpredictability.
This does not mean slowing down AI development—the progress is indeed amazing and should be celebrated. But as these systems become more sophisticated and as debates about AGI continue, we need to maintain a balanced perspective that recognizes both their transformative potential and their fundamental limitations. The future of AI is not about achieving perfect understanding or control, but about learning to work effectively with systems that, like our own brains, may always retain an element of mystery.
Aaron Andalman is the chief science officer and a cofounder at Cognitiv.
The opinions expressed in Fortune.com commentary pieces are solely the views of their authors and do not necessarily reflect the opinions and beliefs of Fortune.